The "deluge of crappy papers" must stop
There should be repercussions for scientists who pollute the commons with crappy papers
A recent paper in the Proceedings of the National Academy of Sciences called “Slowed canonical progress in large fields of science” analyzed 90.6 million papers to study if the exponentially increasing volume of publications is impeding scientific advancement and innovation. Here’s how the researchers summarized their findings:
“Examining 1.8 billion citations among 90 million papers across 241 subjects, we find a deluge of papers does not lead to turnover of central ideas in a field, but rather to ossification of canon. Scholars in fields where many papers are published annually face difficulty getting published, read, and cited unless their work references already widely cited articles. New papers containing potentially important contributions cannot garner field-wide attention through gradual processes of diffusion. These findings suggest fundamental progress may be stymied if quantitative growth of scientific endeavors—in number of scientists, institutes, and papers—is not balanced by structures fostering disruptive scholarship and focusing attention on novel ideas.”
I call this the deluge of crappy papers. A recent article in Nautilus comes up with a more visually-arresting term - “zombie science”. This is apt, because zombies eat brains. Zombie science also consumes brains, or specifically, brain’s attention. Scientist’s time is one of the most precious resources we have and more and more is being wasted conducting, writing, reading, and reviewing extremely low quality work. People have long talked about problem of academia’s “publish or perish” culture incentivizing “minimal publishable units”, but only now are we realizing the full scale and scope of the problem.
Part of the problem with zombie science is that it is hard to distinguish from rigorous, quality science. The devil is really in the details. The precise details of what makes something zombie science are field-dependent, but here are some high level features:
Shoddy or outdated methods.
Cherry-picked results and/or p-hacking.
No pre-registration.
Lack of blinding and/or randomization.
Improper controls.
Low statistical power / low signal to noise.
Major conflicts of interest.
Small sample sizes.
Fraud.
Invention of unnecessary terminology.
Use of math to impress rather than illuminate.
Extremely incremental work.
The deluge of crappy papers phenomena is closely related to the replication crisis and has been discussed in fields ranging from machine learning and AI to ocean acidification.
As the Nautilus authors explain, zombie science reached new heights during the pandemic as researchers looked to capitalize on the situation to stuff their CVs by pushing out crappy papers. Editorial standards were lowered as journals looked to fast-track articles related to COVID-19. University PR departments were happy to breathlessly cover any research on COVID-19 no matter how poorly conducted and inconclusive it was.
The problem of crappy COVID-19 research extended across nearly every field of science. I previously wrote about the flood of COVID-19 related research within the field of medical imaging, much of which was obviously useless (there is little point detecting COVID-19 in medical images when everyone is getting a PCR or antigen test anyway). Biotech blogger Derek Lowe wrote about the flood of low quality works in drug discovery in his post “Too Many Papers”. As he explains, hundreds of “X for COVID” papers appeared on preprint servers and in low-tier journals, many of which were in-silico studies on drug binding/docking which are known to not translate well to insvivo work. A search I conducted for “curcumin+Covid-19” on Google Scholar yielded 6,800 papers published between 2020-2022. A recent review on curcumin for COVID-19 found 78 relevant studies, only 6 of which could be included because the rest were too poor quality to even properly evaluate. If you read the Wikipedia article on curcumin, you’ll get a sense why this is absurd - curcumin has been long touted as an anti-inflammatory and miracle drug even though it has very low bioavailability and no large scale RCTs to support it.
Retraction watch keeps a list of COVID-19 papers that have been retracted, which now stands at a whopping 213 works. The deluge of crappy papers led to a deluge of crappy indiscriminate reviews too. A systematic review of reviews looked at 280 COVID-19 review articles published during the first five months of the pandemic and found that “only 3 were rated as of moderate or high quality on AMSTAR-2, with the majority having critical flaws”.
Professor John P. A. Ioannidis, the author of “Why Most Published Research Findings Are False”, is first author on a new preprint “Massive covidization of research citations and the citation elite”. As Ioannidis et al. explain, citations patterns have shifted during the pandemic towards more recently published works on COVID-19. This is potentially a bad sign, since recently published work has had less time to be subjected to criticism and scrutiny. Historically most papers receive few citations in their first year, and then only slowly accumulate citations over the course of several years. COVID-19 work, by contrast, has received an unusually high number of citations very quickly, luring in more researchers to work on COVID-19 related projects. Typically, the number of citations a researcher gets correlates with their lifetime number of citations (r = 0.46), but this was found to be not the case for COVID-19 works (r = -0.03). According to Ioannidis, these new citation trends may ultimately disrupt the existing scientific hierarchy and create a new “citation elite”. Whether this disruption will be a positive change is unclear. On the one hand, paradigm-busting scientific advancement is constantly impeded by an elderly elite that is protective of their established interests and generally set in their ways, so disruption of that elite should be welcomed. On the other hand, if the new elite is rising to prominence for the wrong reasons then we will not have the best people leading science in to the future.
The deluge of crappy COVID-19 papers has had many negative effects on humanity more broadly, beyond just consuming inordinate amounts of researcher time and energy. The first major of example of harm from crappy papers during the pandemic was the case of hydroxycholorquine (HCQ). The first paper on HCQ came from the lab of the now disgraced scientist Didier Raoult and was published as a preprint on March 20th 2020. It was accepted into the International Journal of Antimicrobial Agents, where one of the co-authors serves as editor-in-chief, after only one day of peer-review. The study was not randomized and contained N=26 patients in the treatment group and N=16 in the control group. Elisabeth Bik published a detailed analysis of the numerous issues with the paper on March 24th. Most notably, 4/6 patients in the treatment group were dropped because they either were transferred to the ICU or died. This was explained in the study, but this fact and other obvious issues did not stop many high-profile figures from breathlessly tweeting about the study as a major breakthrough, most notably then-president Trump. For those interested in a deeper dive, Ariella Coler-Reilly (@AriellaStudies on Twitter) has created “visual abstracts” graphically showing the difference between Raoult et al.’s flawed study and a much larger study (N=821) that found no effect.
The next major case was, of course, ivermectin. At least three early ivermectin studies were found to be fraudulent and retracted. Wading through the morass of low quality works on Ivermectin is now painstaking work - in a subscriber-only AMA Scott Alexander said he spent over 15 hours on his blog post trying to figure out WTF was going on. Further polluting the commons were bad meta-analyses that were largely done on poor quality or fraudulent studies. The funnel plot for the indiscriminate meta-analysis at ivmmeta dot com looks really bad:
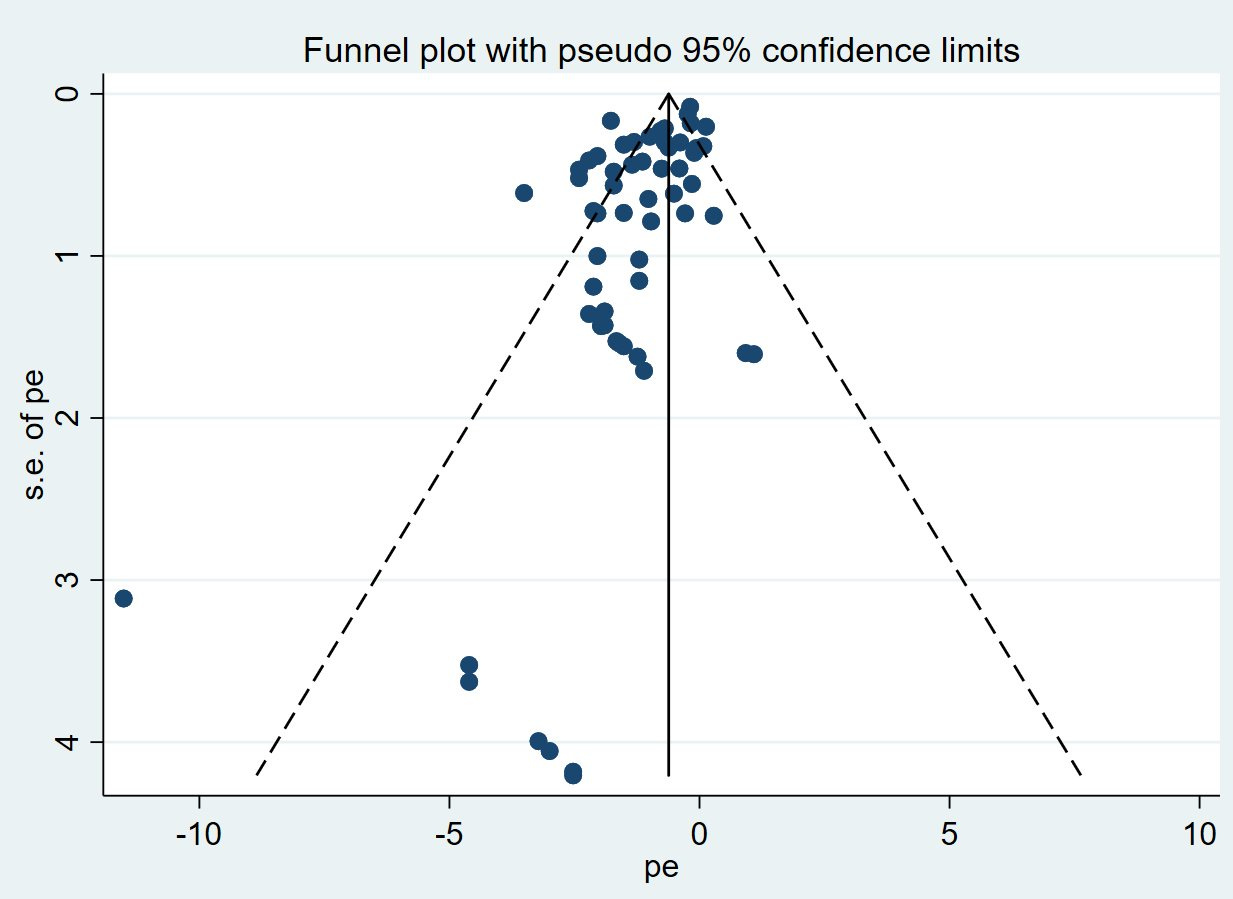
While at least three ivermectin studies were retracted for fraud and numerous more required corrections to be issued, the meta-analyses that included them have not been retracted or corrected. The harm from hype and misinformation around ivermectin has been enormous, especially in countries like Brazil where the government endorsed it as part of their COVID-19 strategy.
Another example is vitamin D for COVID-19, where over a dozen studies on the subject have been either corrected or retracted. Most studies on vitamin D for COVID-19 have been observational studies that are likely confounded. An N=240 RCT on vitamin D conducted in Brazil showed no effect.
How can we fix this mess?
Allow dramatically shorter papers
Instead of getting researchers to cut down the number of papers they write, it might make more sense to reduce the length of papers dramatically. Counter-intuitively we may want to actually encourage the publication of things that otherwise might not be published, such as new laboratory protocols, code, data, or negative findings. This would allow young researchers who are anxious to establish themselves opportunities to publish intermediate work during the course of long (multi-year) research endeavors. A typical PhD student’s typical output might then shift from four low-quality works over four years to one high-quality work and three one-page works.
I’ve done a couple of literature reviews over the course of my academic career, and one what I’ve learned is that the core contribution of most publications can be summarized in just a few sentences. The rest is often just filler. Cutting out unnecessary filler from papers would save time for writers, reviewers, editors, and readers. It could also reduce the time from discovery to dissemination since the process of peer-review and editing would be faster. Right now preprints are proliferating because peer-review takes so long, which is a dangerous trend.
The idea of incentivizing small peer-reviewed papers, also called micropublications, was recently explored at a 2021 NIH workshop. As discussed, natural language processing (NLP) techniques might be used to extract key assertions from both micropublications and existing publications, allowing for easier summary of the literature and the construction of knowledge graphs. Micropapers could also be useful for publishing important results which are hard to fit into existing templates such as negative results, new laboratory protocols, and the development of datasets and code.
Let’s look in a little more detail of how much filler most papers contain:
Introduction
Introductions generally just re-hash well-known fundamentals of a field and concoct some story to justify why the thing study is being studied which probably has little resemblance to the true story. Often content in such sections is lifted nearly verbatim from existing works. A few canonical references are typically cited.
Methods
In light of the reproducibility crisis many have been calling for longer and more detailed methods sections. I am in favor of this. However, current methods sections often contain lots of filler material - when I was working in molecular simulation, for instance, I read dozens of papers that all contained similar background on how molecular dynamics simulation works or how a particular type of quantum simulation method is derived. Likewise, works that use machine learning often explain for the millionth time how a standard CNN works. I suggest that methods sections should be replaced with a list of explicit steps to reproduce the results of the work. Detailed pedagogical digressions are of little utility, especially when there are already well-established pedagogical texts available.
Mathematical theorems
To many papers contains unnecessary mathematical theorems and jargon, including pointless new definitions and notation schemes that confuse rather than illuminate. This is especially the case in the field of AI and in some areas of physics and chemistry. Does anyone actually read all that math? (Fully comprehending mathematics is very time consuming - one hour per page is very fast even for many professional mathematicians). Math for its own sake has no place in science.
Results
Many papers present too many results. Tables with too many rows obscure not illuminate. Most papers have one or two genuinely interesting and valuable finding that motivated the paper and then dozens of incredibly less interesting results. On the other hand it is nice to have antecedent data and ancillary findings available for the sake of reproducibility and transparency, so some sort of balance must be struck. I think it is possible to achieve a good balance though by having the amount of data allowed in the publication strictly limited, but then allowing virtually unlimited data to be presented in the supplementary information.
Conclusion
Conclusion sections generally just contain a lot of material that is redundant with the abstract and introduction. They should be limited to just a few sentences.
References
I am in favor of strictly limiting the number of references. Too many people are “citing to get cited”, similar to how follow-backs work on Twitter. Limiting the number of references forces researchers to identify the most important works that other people should read. A different option would be to somehow create a hierarchy of references whereby key high quality works are distinguished from works of lower quality and importance. Many works currently accrue citations precisely because they were poor quality work that was disproved. Maybe we need a “negative citation” that works similar to a down-vote on Reddit?
Stop funding low-powered studies
Funding agencies need to stop funding under-powered studies using sloppy statistical methods and small group sizes. This will mean that fewer groups will receive funding. However, without some pain there will be no improvement. Labs should be forced to adapt to a harsh new reality that they will only get funding if they conduct high powered, pre-registered, well-designed studies that are analyzed with modern statistical techniques. This may require some university labs to merge to pool their staff and other resources to they can conduct and properly analyze larger studies. Creative destruction may be called for — some labs that have only been publishing crappy work may have to shut down and some professors may have to be forced into early retirement.
Fund longer-term projects and embrace the journal hierarchy
In my experience most graduate students and postdocs publish about one journal paper per year on average across many fields. This rate should probably be much lower. Funding agencies may want to introduce a point system whereby papers in more prestigious journals with higher standards and lower acceptance rates would count much more than papers in lower tier journals. So, one high quality Nature, NeurIPS, or Phys. Rev. paper after four years of work should be treated equality to four low quality works published during the same time period. Papers published in journals on Beall’s list of predatory journals should count in a negative fashion. Hiring committees should also focus on quality over quantity, using journal ranking as a proxy for quality when necessary.
Crack down on fraud, hard
I believe the prevalence of fraud is much larger than is currently appreciated and that fraud is a big reason many studies fail to replicate ( I hope to do a post at some point diving deeper into this under-explored subject). The pressure to publish incentivizes many young researchers to commit “subtle fraud” like massaging or cherry picking data. When fraud is discovered by other lab members, the lab head will exert pressure to make sure it isn’t shared publicly. It goes without saying that all of this needs to stop.
The NSF should have a fraud investigation unit. Imagine how much fraud could be uncovered if the NIH or NSF funded a dozen Elizabeth Biks. Bounties on fraud detection could also be offered. [At some point AI systems should be able to detect fraud as well although from what Biks and others have said we are still quite a ways from that despite a lot of hype and work on it.] Once clear-cut evidence of fraud has been discovered, the labs, offices, and computers of all researchers involved should be ordered locked down immediately and the researchers in question should be bared from accessing them. Labs and offices should be sealed off with yellow tape so everyone knows what is going on. Future funding should be made contingent on the Universities’ full compliance with the fraud investigation. Next, a fraud investigator or team of investigators should be flown out. Being a fraud investigator should be a high status job and carry trappings of status such as badges, uniforms, cool looking suitcases, and first class flights. Researchers thought to be involved should be interrogated in recorded sessions. Then, all evidence found should be presented in special court. Punishments for researchers found guilty of fraud should be severe. For cases where a professor knowingly committed fraud, they should be banned from receiving funding and pressure should be exerted to get them fired. PhD students should be expelled. A national public register of fraudsters should be kept.
Acknowledgements
Thank you to Bonnie Kavoussi (@bkavoussi) for proofreading an earlier draft of this post. Please check out her Substack here.
Like the idea of shorter papers - I can definitely agree with your observation that a big part of most papers could be scrapped without a big problem.
Regarding your last point: I agree that we need to get better at fraud detection, however I am not sure if harsh, public punishment is the way to go about it. As you mentioned there is a lot of external pressure on (young) scientists to engage in "subtle fraud" and also, I believe that a lot of research mistakes do happen not with bad intentions but due to (sloppy) mistakes. In consequence, I was wondering whether it could be maybe more effective to push for a 'failure culture' in science and change the incentive system? This would entail e.g.:
- making it acceptable for someone to correct their own mistakes - if a scientist finds a mistake in his/her own study and corrects it, they should be congratulated and not shamed
- incentivize people to replicate/check each others work - checking other people's work currently isn't rewarded at all in the academic incentive system
- incentivize metastudies and aggregation of multiple studies (which would bolster the current evidence and make it harder for individual fraudsters to go unrecognized)
What do you think about that - curious to hear your opinion!
Good ideas.
It's unfortunately tough to be a young academic these days -- lot of time spent trying to get positions, get grants and turn out papers.
I saw an interview of Peter Higgs a few years ago.... one sec..... ahh here it is:
"Peter Higgs, the British physicist who gave his name to the Higgs boson, believes no university would employ him in today's academic system because he would not be considered "productive" enough.
The emeritus professor at Edinburgh University, who says he has never sent an email, browsed the internet or even made a mobile phone call, published fewer than 10 papers after his groundbreaking work, which identified the mechanism by which subatomic material acquires mass, was published in 1964.
Speaking to the Guardian en route to Stockholm to receive the 2013 Nobel prize for science, Higgs, 84, said he would almost certainly have been sacked had he not been nominated for the Nobel in 1980. Edinburgh University's authorities then took the view, he later learned, that he "might get a Nobel prize – and if he doesn't we can always get rid of him"....."
Howard (Toronto)