Happy 2024! This is a bit different style of post.. more of a string of loose reminiscences mostly written over the summer. Eventually I am planning to post some more personal reminiscences on my Medium, so check that out if interested.
Data “Science” fantasies
While working on my Ph.D., I spent several years staring at an old Dell monitor in a room with stuffy air, cinderblock walls, and flickering fluorescent lights. There were no windows and the floors were vacuumed about once a year. When I finally moved to a new office, the heat didn’t work for months. Still, I worked long hours in that freezing office, periodically going to the bathroom to warm my hands. Everyone around me had mental health issues. Needless to say, I wasn’t too keen to stay in academia.
We were told that getting a job would be easy with a Ph.D. in physics. A life of luxury beckoned—six figure salaries, sunlit offices, fancy coffee machines, and an endless supply of La Croix. We were told that “data scientist” was the sexiest job title in town. In addition to the sex appeal, cash, social status, and bubbly water, many of us were intellectually enraptured by those mysterious entities called neural nets. People were programming miniature brains into computers and then watching them predict the stock market or find a cat in a photograph. Nobody knew how they worked. The world was waiting for the next Newton or Einstein to unravel it all. In the process the secrets of the brain, and perhaps even consciousness itself might be unlocked. This was the new frontier of science.
The first time I realized this dream life might not be all that it appeared I was at a workshop in early 2016 on how to transition from a Ph.D. to industry. A young woman was the only speaker at the event who actually worked as a Data Scientist—the rest were all career coaches. Someone asked her what her day-to-day work looked like. “Well,” she said, “most of my day I’m fixing Excel spreadsheets and getting data formatted correctly.” You could almost hear the sound of multiple crests falling in synchrony. She went on: “I love my job, though, I really do. I go in the same time and leave the same time every day. My boss is really nice. I have more time for my friends and family. It’s a really great life.”
It didn’t sound very convincing.
Today, about half my graduating class of physics Ph.D.s work in so-called data “science.” Great minds that used to ponder the ultimate fate of the universe or invent revolutionary new types of materials now spend their days doing grunt work like cleaning data, stuffing code into Docker containers, and debugging issues with Python library version numbers. Every Ph.D. I talk to who went into data science says their days are filled with boring menial work. Still, they often like their job due to the nice offices, good pay, friendly co-workers, and amazing work-life balance.
It turns out it wasn’t easy to get a job in data science, even with a Ph.D. I had no experience with machine learning. Most of the jobs I found involved customer relationship management, targeting ads, or detecting fraud. None of these topics interested me. I also began to realize that “neural networks” were not that intellectually exciting, either. Do linear regression, and then set all the negative numbers to zero. Repeat that a bunch of times. That’s a “neural” net. No spiking, no recurrence, no online learning, very little resemblance to anything in the brain.
I also learned there’s no actual “science” in “data science,” unless you consider fitting curves science. I did not and still do not consider that science, because science is about deep understanding and finding what David Deutsch calls “good explanations”. Anyone can fit a curve. Enrico Fermi used to say “with four parameters I can fit an elephant, and with five I can make him wiggle his trunk”. Good explanations, however, are hard to find. Good explanations reach beyond the places they were born and lead to insights that result in new inventions and discoveries. Good explanations extrapolate, while data science can only interpolate. Using data “science” methods to make predictions is a perversion of what science is all about.
AGI or bust - the Mindfire AGI moonshot
In 2018 there was a short-lived push to create artificial general intelligence (AGI) called Mindfire. It was initiated by Swiss entrepreneur Pascal Kauffman. The idea behind Mindfire was to bring together experts from many different fields to build AGI. They had a very ambitious roadmap which aimed to create AGI in four years:
A diverse group of computer scientists, hardware engineers, data scientists, AI researchers, and neuroscientists were all flown to Davos, Switzerland. The meeting was held in the Intercontinental Hotel, which is famous for as a key location for the World Economic Forum.
The event kicked off with a reception. Everyone had an “angle” on how to create AGI. A few of the people there were genuine crackpots. Greying hair, grizzly beards, and a desperate light in their eyes. They were weary of life but believed they had hold of one idea that would change everything. I received my first crackpot pitch about a half hour into the welcome social. “The brain is a dynamical system!” it began. The brain, I was told, operates at the edge of criticality, where self-organizing bursts neural activity orbit dynamical attractor states. Intelligence, I was told, is a complex emergent phenomena that could be reproduced by just a few coupled nonlinear differential equations. Inundated with such a prolific amount of inchoate techno-babble, I could only nod my head and mutter a few points of agreement where I was able to.
The next crackpot couldn’t stop talking about how linguists had been persecuted and marginalized from the AI community. The current approach of feeding big data into transformers like BERT was all wrong I was told. Those promoting BERT were just trafficking in superficial statistical correlation. The truth, I was told, is that language has a hidden structure which had been unlocked by great linguistic theorists in ages past. Sadly, though, AI people lacked the ability to comprehend such arcane knowledge, so they turned away, pretending it didn’t exist. But there was hope – this man did understand the laws of universal grammar and he was going to program them into a computer, giving birth to the first true reasoning engine, a baby AGI from which the singularity would usher forth. There was just one problem, however—he needed someone to give him money to complete this work.
The final crackpot couldn’t stop talking about embodiment. This wasn’t just your run-of-the mill embodiment fan, though. This guy took it to a whole new level. What we needed to do, he said, was to build robots and have them explore on their own in the wild. We had to release them into nature, to live among the animals. The AI must run free in nature, exploring rocks, streams, and all that it has to offer. Yes, it would be hard to survive in the wilderness, but it would learn faster that way.
The rest of the day was spent eating very expensive food and drinking expensive wine as we admired the mountain views. At some point the mayor of Davos showed up. Davos was going to be an AI city, we were told. AI developers would be lured by the beautiful mountain vistas and clear babbling brooks. It was a nice thought.
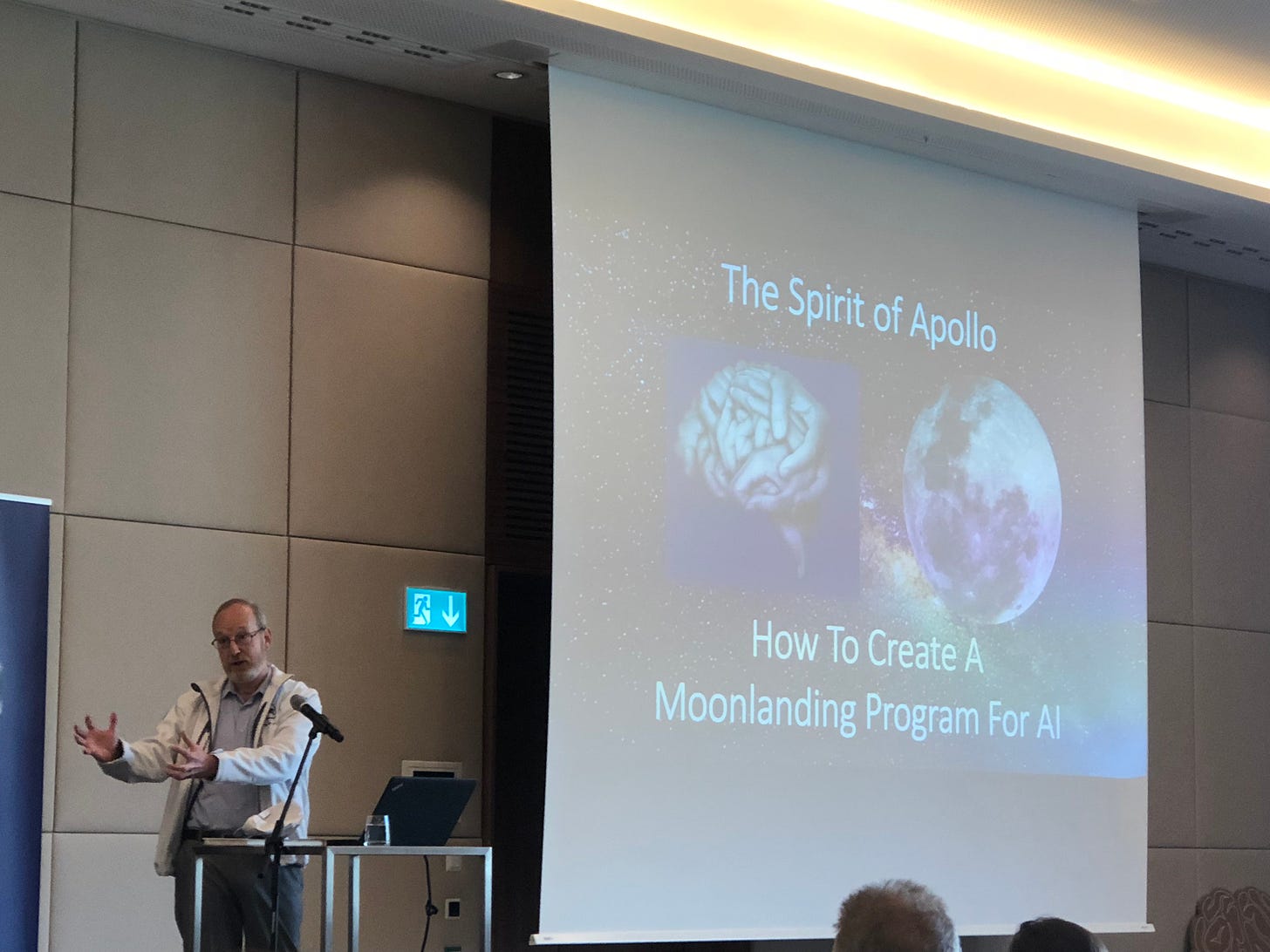
After a lavish four course dinner, lightshow (yes, a lightshow) we headed back to our hotels to sleep. We woke up the next morning ready to roll up our sleeves and build AGI. First, however, we had to do some ice-breakers to get to know each other. So, we went to downtown Davos and did a treasure hunt, getting clues and walking through the city. That wasn’t enough though, so we also did an escape room. After taking a bus back to the Intercontinental, then it was time to build AGI.
We all were seated in teams and asked to brainstorm our approach. The table fell silent. Nobody had any concrete ideas on how to build AGI. I suggested that maybe we might need different modules—for instance a perception system for processing inputs, a world modeling system, a planning system, and a motor control system. I drew a diagram of how I thought the modules should be wired together. Later, after presenting this plan to the assembled audience, I was told by one of the organizers that I was “not being creative enough.”
Then, Ben Goertzel made a surprise appearance. The energy in the room was palpable. He pitched the idea of AIs living on the blockchain, exchanging services. He mentioned hypergraphs as the key to AGI. Later I was talking to a prominent German neuroscientist He found it suspicious that Goertzel never explained what a hypergraph was. He asked me if I knew. I did not.
Everyone left the conference energized. But then nothing happened. It turns out building AGI is hard, and academics are too busy chasing publications and grants to volunteer their time for such an effort. Davos did not become an AI city and I never got my Mindfire crypto tokens.
Better molecules through AI
In 1898, the German chemist Georg Friedrich Henning patented a new drug called cyclotrimethylenetrinitramin (try saying that 10 times fast, or even one time). It was a lousy drug, but it didn’t take long for German chemists to realize it was also an explosive 150% more powerful than TNT. During WW2 the Allies developed it further. They called it “Research Department eXplosive” or RDX, and they started producing it in massive quantities. RDX helped the Allies win the war against the Nazis. Since WW2, RDX has been the most used military explosive molecule in the world. Ever heard of C4? It’s actually just RDX, with some plasticizers added in.
For 70 years chemists have been searching for an alternative however, for RDX is nasty in several ways. First, RDX is highly carcinogenic. Wildlife that consumes bits of RDX dies. Over 65 military installations are currently contaminated with RDX. RDX is also a potent GABAA receptor antagonist, a sort of inverse Ambien. Soldiers exposed to RDX experience intense seizures.
RDX also has another dirty secret too—it sometimes explodes when people don’t want it to. There are anecdotal stories of RDX bombs falling onto aircraft carrier flight decks and spontaneously exploding. The Navy has no idea why this happens, although it is believed to be due to defects that have accidentally been introduced during the manufacturing process.
For decades the US military has funded synthetic chemists to find a safe and environmentally friendly explosive—the ultimate oxymoron come to life. By 2016 most of the US’s synthetic chemists who have worked on the problem were retiring. Meanwhile, in China there were dozens and dozens of chemists working on the problem, publishing papers at a prodigious rate.
Enter our crack team at UMD. Through the magic of AI, we were going to beat the Chinese and solve this problem that had alluded chemists for seventy years.
A major story was In Silico Medicine’s use of AI to find a DDR1-kinase inhibitor that did well in mice. The entire process took only 46 days. I’ll spare you the technical details.1 The story of this achievement was widely publicized in the press. It appeared that the drug discovery process was just sped up from years to months. Everything would be done by AI. AI would be better than pharmaceutical chemists at designing drugs that were selective, non-toxic, and bioavailable. AI was going to be better than synthetic chemists at designing synthesis procedures. AI would design clinical trials. Basically, AI was going to do everything!
What few people realized though (at least not right away) was the In Silico “breakthrough” was largely a mirage. Firstly, the “46 day” development time didn’t take into account the time required to develop custom reward models for the specific task at hand. Those reward models were essential for implementing reinforcement learning. Even more important is the fact that the final molecules were very similar to existing drugs that were developed for similar application.
We ran into something similar. Our AI (which was a special generative network) generated a lot of plausible explosive molecules. However, many were just variations around a couple known molecular skeletons.
The AI will see you... uh, once we work out these bugs…
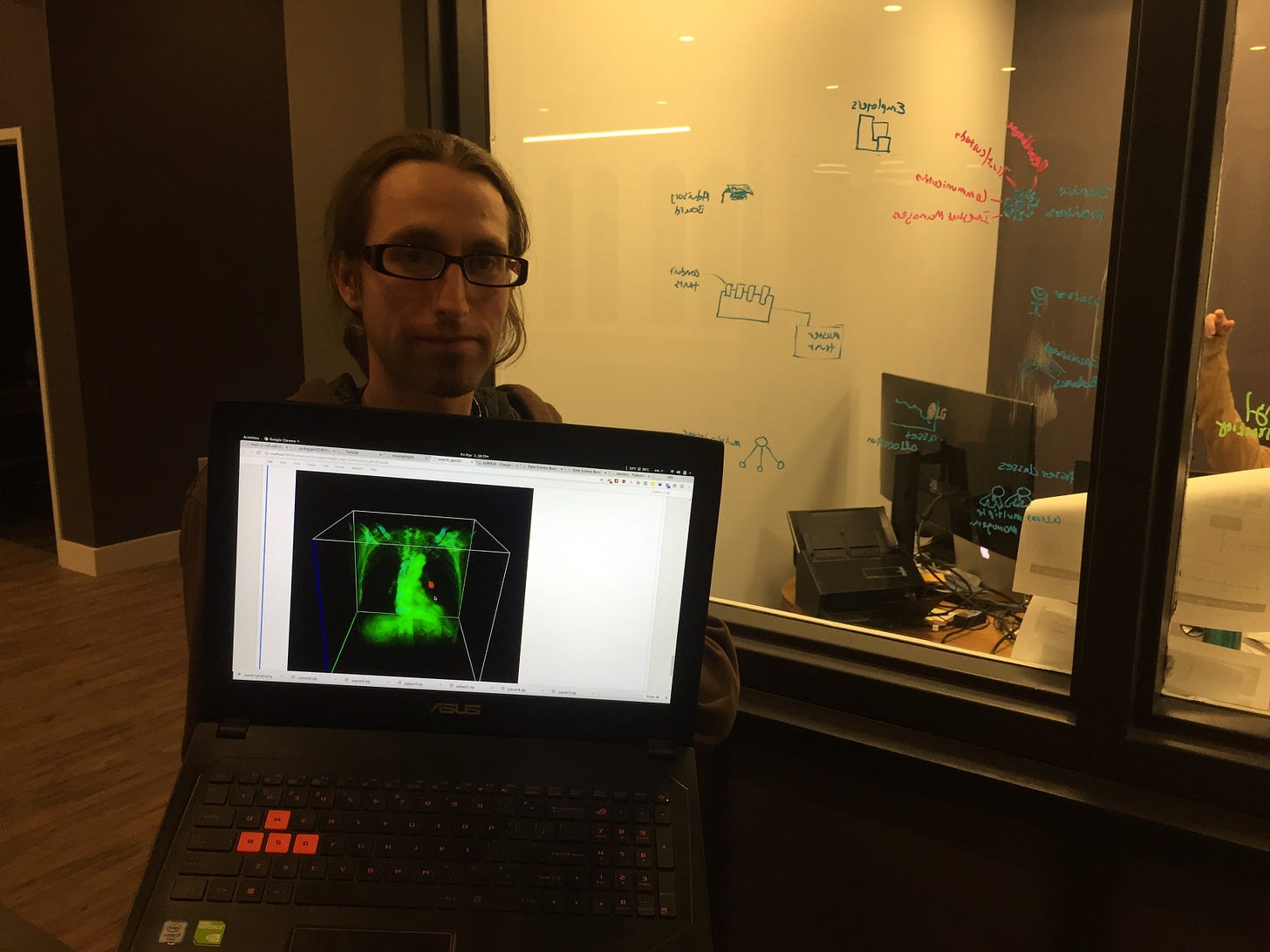
In January 2019 I started a new job at NIH working in the lab of Dr. Ronald Summers. Our focus was on AI for radiology. I did a lot of great work there, mostly on automating measurements of organ volume. I learned a lot there. Mostly I learned that it’s really hard making deep learning models robust. They need ridiculous amounts of carefully labeled data, which is expensive to procure for medical images. Even then, deep learning models may fail in utterly unexpected and unexplainable ways..
In 2016 Geoffrey Hinton had famously declared that we should “stop training new radiologists” and that deep learning would be “as good as radiologists” in 5-10 years. I bought into that notion at the time. Once I started working in the field, though, my view changed dramatically. I wrote about all of this in my March 2022 article “AI for medicine is overhyped”, which is by far the most-read thing I’ve ever written. The article is mostly about the lack of robustness of AI systems, even commercialized ones, and the difficulty of deploying AI into hospital environments. I don’t recount all that here. Instead, I’ll just give a birds-eye overview of the rise and fall of what deep learning for radiology.
After the deep learning revolution of 2012 there was explosion of AI for healthcare startups. I tried Google searching some of the names on the above graphic and many of these companies have gone out of business. I suppose that is expected for startups in general, though.
Many of these startups concentrated on radiology. (Pathology would be another prime target, but most pathology departments still use old fashioned light microscopes and haven’t even digitized yet!)
The explosion of AI for healthcare startups continued to grow until at least 2020. During the pandemic academics churned out thousands of papers on “AI for COVID” papers, almost all of which were utter crap, as I wrote about in August 2021.
In early 2022 IBM sold off “Watson Health”. They had invested over four billion into it, but sold it for pennies on the dollar. Then in late 2022 tech stocks started to tumble. Investment into healthcare AI also dropped. Then, investment continued to drop even as tech stocks have recovered.
Most AI for healthcare startups have gone bankrupt now. Off to the right of the above graphic I put some AI for radiology startups which are considered leaders. These companies make products with genuine utility. However, most hospitals can’t afford the cost of the AI systems they sell. Hospitals also don’t have the staff needed to properly deploy, integrate, and monitor AI. Insurance companies won’t cover AI right now, either, except in one or two specific cases.
In retrospect, applying the machine learning and deep learning paradigm to healthcare was never going to work. To replace a radiologist, you’d have to have thousands of separate ML models. Orchestrating all of those models and making sure they receive the right sort of imaging data would be a massive headache. All of those models would have to be trained on massive, diverse, carefully labeled datasets, which are very expensive to obtain. Furthermore all of the models would have to be continually updated since healthcare is rapidly evolving, which is logistically difficult (and only supported to a limited degree by the FDA).
The future lies in foundation models - a single model trained in an unsupervised fashion on large amounts of images and text from hospitals. Which brings us (finally) to the conclusion of this post..
Is this time around different?
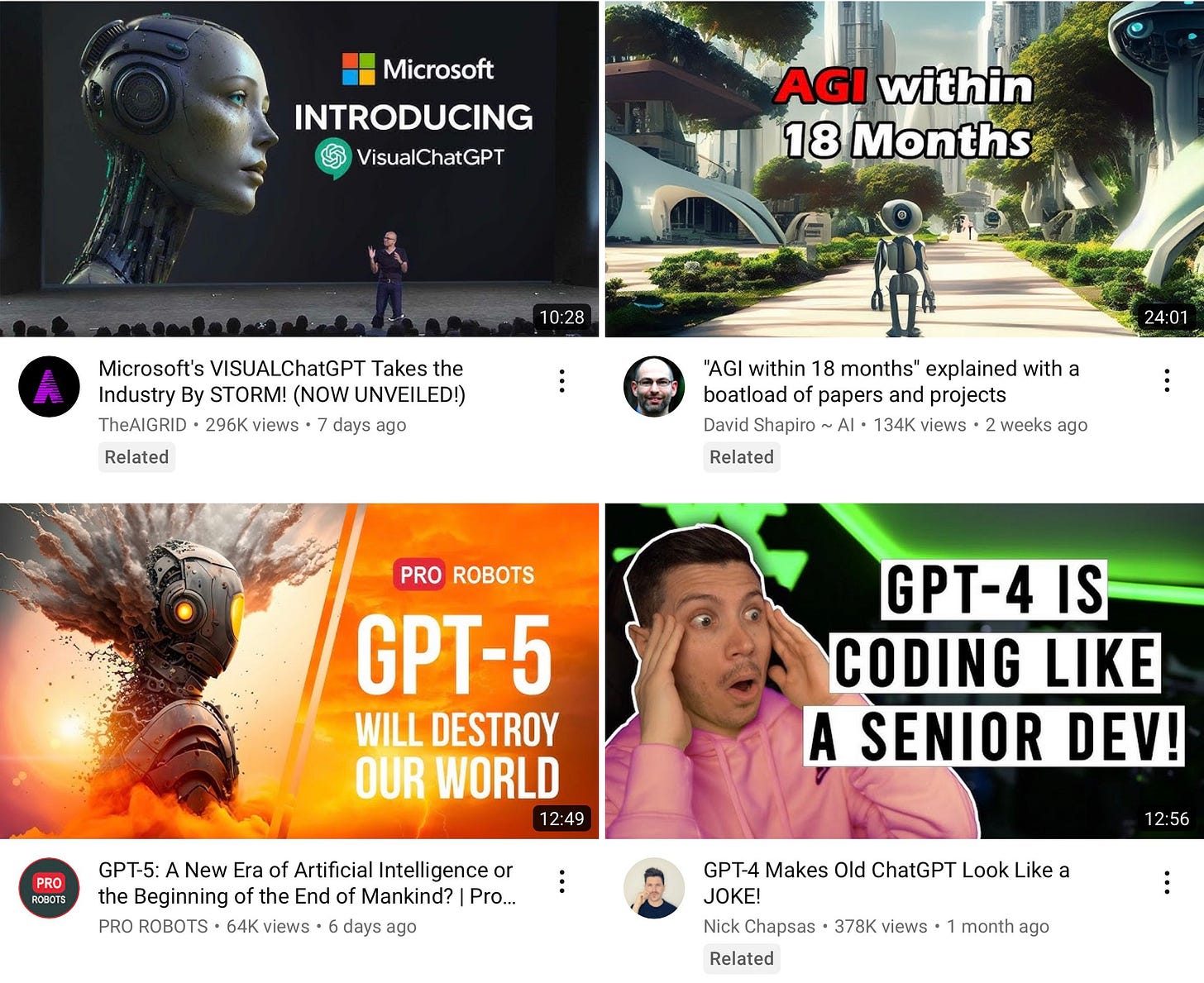
The latest wave of AI hype was triggered by the release of ChatGPT about a year ago. It’s called either the “generative AI” wave or the “foundation model” wave.
Is this time different? Well, it certainly feels different.
Let’s zoom out and take a bird’s eye view at the history of AI:
1956 - 1974 - 1st AI wave (birth of the field) (18 years)
Dartmouth conference (1956), Perceptron (1957), ELIZA (1965)
1974 - 1980 - 1st AI winter (6 years)
1980 - 1987 - 2nd AI wave (expert systems) (7 years)
LISP machine (1980), Boltzmann machine (1985), PDP paper (1987)
1987 - 1993 - Second AI winter (6 years)
1994 - 2011 - Steady progress (development of machine learning)
LSTM (1997), Lecun’s CNN (1998), ImageNet (2006)
2012 - 2021 - 3rd AI wave (deep learning)
GPU training of deep CNNs (2011 - 2012), Batch Norm (2015), Deep RL, GANs, BERT, GPT-2
2022 - present - 4th wave (generative AI)
ChatGPT, scaling laws, stable diffusion, RLHF
The big thing I’ve gotten out of this cursory review of AI history is that the “major boom followed by major winter” trend hasn’t really held since ~1994. Since then, it been more like “steady progress but with a couple major jumps”. Obvious jumps are the GPUs for training in 2012 and the release of ChatGPT. Ignoring the jumps, the underlying trend recently has been tracking pretty well with Moore’s law.
While there is no doubt AI has been advancing the last ten years, and the rate of advancement is accelerating somewhat, that doesn’t mean AI isn’t over-hyped. The hype has also accelerated. People are talking about “AGI” by 2030, massive disruptions to the job market, and movies being created by AI soon. As I will discuss in an upcoming post, LLMs are very useful tools, but they still are fundamentally flawed in many ways. Even if scaled up further, I don’t think they bring us much closer to truly transformative superintelligent AGI. There will be a post on this soon!
The researchers used a custom generative adversarial network and reinforcement learning to generate 60,000 molecules. They then randomly selected 40 structures that spanned the chemical space and used a large collection of established heuristics from synthetic chemistry to narrow this pool further to just six molecules, which were tested in cell culture. The most promising candidate was then tested in mice.
I generally agree with you and find your perspective valuable. One thing that might be useful is to point out where you disagree with a few play money prediction markets on AI trends. Eg I "bet" against this awhile ago: https://manifold.markets/ScottAlexander/in-2028-will-an-ai-be-able-to-gener. I find it extremely unlikely that Hollywood is going to be automated within the next five years.
Dan, great article! I really value the retrospective.
This truism pains me: ".Every Ph.D. I talk to who went into data science says their days are filled with boring menial work. Still, they often like their job due to the nice offices, good pay, friendly co-workers, and amazing work-life balance"